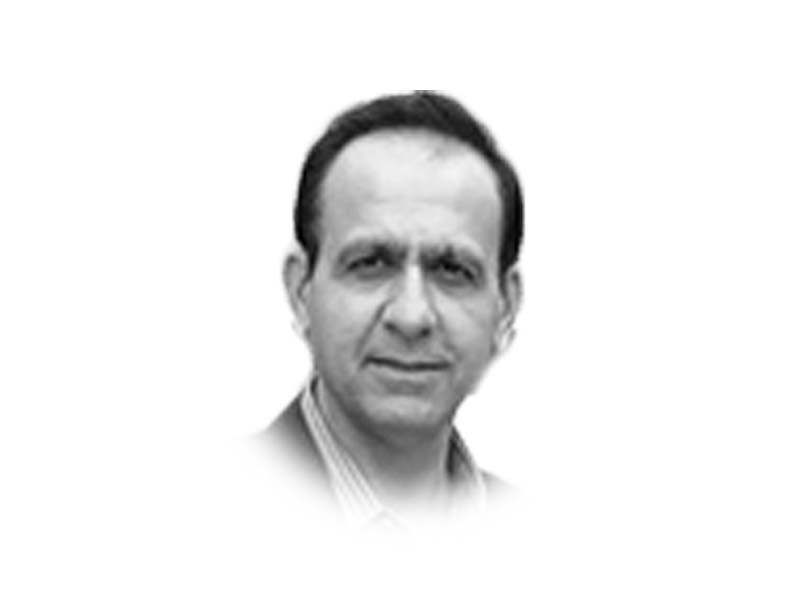
It was many years later that we are exposed to different types of curves and the relationship they represent between the x and y values. The simplest are the linear relationships which are plotted on a straight line primarily because the growth in y is proportionate to the rise in x. However, we soon realise that the most interesting relationships are not linear. For instance, exponential growth of y occurs when its value climbs astronomically faster than the rise in x.
Exponential growths can be extremely rapid, so fast that even the universe is scientifically believed to have grown at that speed. The theory of inflation in the field of cosmology details the period after the big bang that resulted in an exponential expansion of the universe and eventual creation of milky ways, galaxies, and many other celestial bodies.
Amongst the examples of exponential growth, it might be surprising for some readers if evolution was mentioned as one of them. This is because historically speaking, the process of natural selection is perceived to be a “slow” procedure, although this view should have been refined when John Holland, one of the principal researchers and founder of genetic algorithms, published the schema theorem in 1975. The ‘70s were the formative years of genetic algorithms — an AI based methodology inspired by the theory of natural selection and survival of the fittest. Holland’s schema theorem uncovers an elegant exponential relationship that describes the workings of genetic algorithms, and by association, this formula can be generalised to broadly cover the scope of natural selection. In short, powerful exponential functions that underpin the speedy expansion of the universe also explain the effectiveness of the genetic algorithm and evolution.
The schema theorem marks a watershed moment in the understanding of genetic algorithms as well as evolution in general. This was because prior to the schema theorem, despite the growing body of evidence, there was very little research that mathematically explained the mechanics of natural selection and why it worked so well.
The application of genetic algorithms to real-life problems can be explained through a simple example of a hypothetical clothing shop. Imagine the shop sells only three types of shirts: A, B and C. After a sales period, let us suppose that design A is selling the most, followed by design B, whereas design C is struggling to gain minimum traction. For the next sales cycle though, instead of simply ordering from the existing designs, the management could choose to introduce new designs based on the designs that were previously popular, A and B. If every design can be described in terms of their subcomponents, we could combine and merge them together to create more designs. The subcomponents could be items like the type of fabric, its colour, collar and cuff design, etc. Intuitively, this idea seems to have merit, since we are assimilating two most popular designs to create more variety. However, if in the process of this experimentation, a particular new design does not do well, it could be given less priority subsequently in line with what happened with design C.
Hence, one can establish a mapping between the above process and the concept of survival of the fittest. Every shirt design could represent an individual, whereas the subcomponents of the shirt are its respective genes. The fitness of an individual is measured by its sales and the mating or recombination is more likely to happen between successful designs. Obviously, the above is a simple example to demonstrate the concept. In real life, genetic algorithms are applied in situations that are far more complex for humans to resolve manually.
From the perspective of an external observer, the process of evolution results in the rapid multiplication of species that adapt with time. However, what the schema theorem explains is that if we were to take into consideration the entire population, the cluster of genes that are the fittest also drastically enhance their count in each new generation. Holland called it implicit parallelism which means that although explicitly the adaptive species dominate implicit within this process is the exponential and simultaneous rise of all the patterns of genes from the fittest individuals. It is therefore the repeated combination of these dominating gene patterns in multiple ways that result in generating better and better instances.
The application of the schema theorem is likely to rise substantially as scientists uncover the role of evolution beyond biology. Richard Dawkins introduced the concept of memes which are akin to genes and represent a unit of cultural behaviour or idea. Since cultural practices consist of memes and as the dominating ones tend to pass down through generations, he postulated that these undergo evolutionary process as they meet all the necessary preconditions for it. Dawkins meme theory is just one of the many such social, economic, and cultural theories that explain the evolutionary transformation of ideas and practices within societies.
As evolution increasingly explains various non-biological processes impacting our lives, the schema theorem details the underlying mechanism of how these societal transformations take place. It is fascinating that a theorem that was initially formulated to describe the process of genetic algorithms has achieved truly global influence, relevance, and applicability.
COMMENTS
Comments are moderated and generally will be posted if they are on-topic and not abusive.
For more information, please see our Comments FAQ