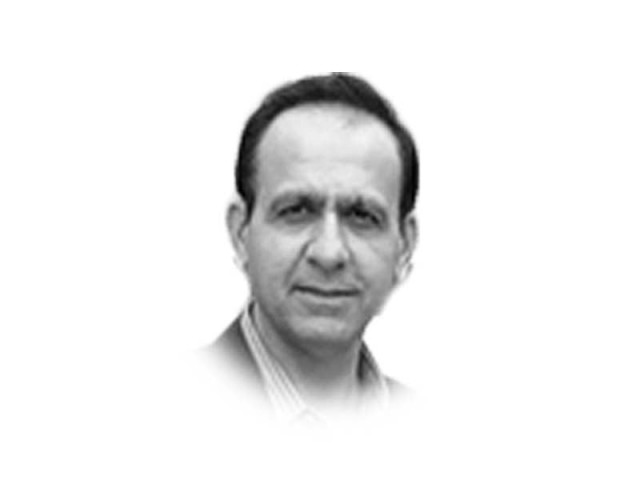
The prevailing mantra within organisations is to encourage employees to report failures. This is in line with the belief that considers failure as an important platform of learning which has hitherto been ignored in favour of receiving accolades for successful outcomes. Whereas a failed project in the past was a sure sign of being shown the door by the management, the mindset has evolved to value employees that are keen to experiment despite failing at times to implement innovative practices.
Although there can be no denying the importance of learning from failures, it is most effective in conjunction with our generalisations from successful outcomes. Research in artificial intelligence and machine learning indicates that the nature of what is learnt from success is fundamentally different to the nature of learning due to a failed result. Therefore, contributions from both successful as well as unsuccessful experiences are essential prerequisites to achieve impactful learning.
Learning from labelled examples of success and failure is also referred as inductive learning. One of the earliest inductive learning AI programmes was developed by an MIT scholar by the name of Patrick Winston in 1970. His system automatically learnt the concept of an arch when presented with categorised positive and negative cases. For instance, a positive example consisted of a horizontal brick placed on top of the two vertical bricks, whereas, one of the negative examples was identical, except with no gap between the vertical bricks. Therefore, given a series of positive and negative cases, the system continuously refined its definition to achieve a better understanding of an arch.
Winston’s machine learning programme, along with other studies that followed, aptly demonstrated the different types of learning that take place in lieu of positive and negative stimulations. The research shows that when presented with a positive instance, the inductive learning algorithm stretches the target definition by making it broader. For example, when the system was presented with a positive instance of an arch that had, instead of a brick, a wedge placed horizontally on top of two vertical bricks, it automatically refined its definition of an arch by relaxing the condition for the horizontal brick to be either a wedge or a brick.
Hence, this widening of the definition of an arch with every positive instance is a form of generalisation. Interestingly, it is not difficult to work out that if we feed the system with mostly positive cases, the definition of an arch over a period will become over-generalised to the extent of becoming vague. This will result in a situation where anything that even remotely resembles an arch will be incorrectly classified as such!
On the other hand, when the system encountered a negative instance, it made the internal definition of an arch more specific. For example, when a labelled negative case of an arch was presented with no gap between vertical bricks, the system firmed up its definition and made the gap an essential condition for its description of an arch. Therefore, if presented with mostly negative cases, the system would wrongly develop a very narrow definition of an arch which would misclassify even the most obvious of arches.
It is evident that a predominant tilt on either positive or negative cases leads to ineffective inductive learning system. To reach a correct understanding of an arch, the algorithm had to take into consideration balanced observations of both types of instances. At each stage, when presented with a positive case, the algorithm enhanced the arch definition to become more inclusive, whereas, the negative examples restricted and made it more exclusive. Eventually, it was the refinement of the target definition (arch) through both generalisation and specialisation whilst evaluating a series of positive and negative examples that led to effective learning.
Although both positive and negative examples are important for inductive learning, historically speaking, businesses have not treated these two types of experiences equally. Whereas successful outcomes have been acknowledged and rewarded by senior management, failed instances have often been a source of shame for employees and had resulted in internal isolation, transfer or termination. As positive outcomes are celebrated and shared across the industry, staff members are constantly bombarded with best practices, and success stories. This enhanced consumption of wins by the employees with relatively few discussions on losses causes over-generalisation of learned knowledge. This is akin to providing substantially more positive and very few negative examples of arches within the context of Winston’s machine learning programme.
The direct effect of over-generalisation is that professionals become prone to making mistakes as they frequently misclassify new business situations and apply incorrect solutions. Interestingly, to an observer, such employees may also falsely appear agile and confident since over-generalised learning makes them wrongly believe in their ability to respond. Overall, therefore, the net result for a firm is an increased probability of business risk due to employee errors.
Hence, the current drive by organisations to motivate sharing of failures is prudent as it can create a healthy equilibrium between exposure to successful and unsuccessful cases. However, as businesses push towards promoting learning from failures, they should guard against propagating high volumes of unsuccessful outcomes to employees. This is important since if this is not managed well, the workforce could traverse towards an equally harmful opposite direction where their learned knowledge becomes over-specialised. In this case, the situation will be compatible to an instance of Winston’s programme which learns from many more negative and fewer positive examples of arches. Therefore, it is crucial that a parity is maintained between broadcasting of wins and losses to enable organisations to reap maximum benefits from its history and experiences.
In an ideal setup, learning from victories should help us expand our horizon and provide insight into the art of the possible. Whereas, every instance of a loss should help us learn to avoid mistakes when we encounter similar situations. Achieving excellence, therefore, is only possible through a holistically learnt knowledge built upon the pillars of both our successes and failures.
Published in The Express Tribune, July 14th, 2019.
Like Opinion & Editorial on Facebook, follow @ETOpEd on Twitter to receive all updates on all our daily pieces.
COMMENTS
Comments are moderated and generally will be posted if they are on-topic and not abusive.
For more information, please see our Comments FAQ