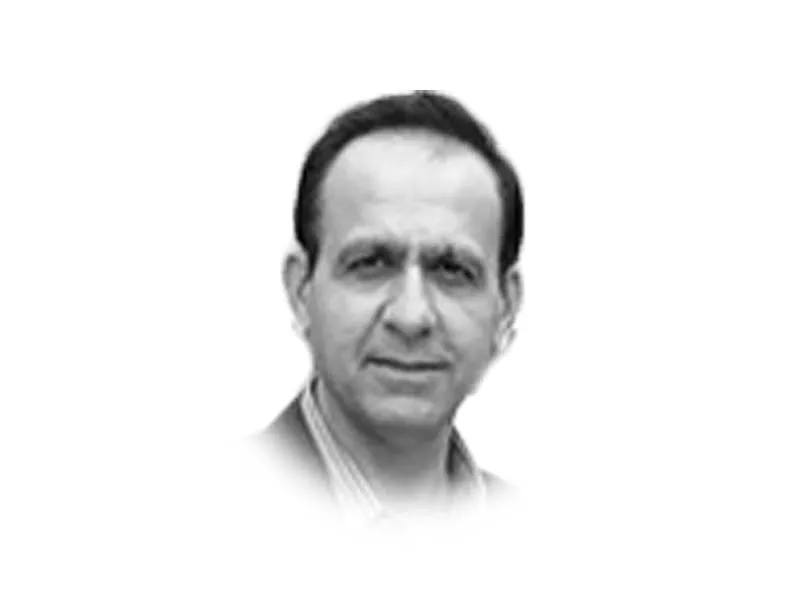
As a child it was fascinating to observe ants marching back and forth in uniformity towards a food source near their colonies. However, back then little did I know about the amazing mechanics that lets ants achieve foraging efficiency. For the ants, a high level of navigational competency is crucial since goodies lying idle for a long period could attract other deadly contenders. Nevertheless, what seems remarkable is the process of interaction between ants that results in solving a genuinely complex problem of route minimisation. In short, an ant’s discovery of an efficient trail follows a process of recombination of useful paths previously treaded by others. This unique process of optimisation has been simulated as an algorithm within the field of artificial intelligence, and it has successfully been applied to solve many real-world problems.
The initial group of ants usually begin their meal excursions by trying out diverse routes that are guided by their incredible sense of smell. This foray is somewhat random in which they often cross paths, avoid obstructions, and discover new ways to reach their desired location. During these explorations, every ant marks its trip with a secreted substance called pheromone. Its main characteristic is a distinct odour that is detectible by other members of the ant colony. As the number of expedition increases, the terrain starts to get crowded with zigzagged tracks. At some stage, the newer cohort of ants begins to try out previously travelled routes by dovetailing its pheromone. However, as an ant ventures on a previously traversed path, it also comes across sections that have been crisscrossed by trails made by other fellow ants. At those junctures, it faces the dilemma of whether to continue with its ongoing trip or change its course by switching to one of the intersected passages to reach the eventual destination.
The ants tend to resolve these deadlocks by following a simple rule throughout their journey. At any point of intersection, they always pick the trail with the highest intensity of pheromone scent. Intuitively speaking, this approach has merit since both shorter as well as popular tracks will tend to accumulate higher levels of smell. Hence, when it encounters a junction with a higher odour intensity, it is quick to disband its original route and hop on to the new course.
However, this switch is also not permanent since if it now encounters another trail with a higher pheromone smell, it rapidly makes yet another transition. The main feature of this greedy approach is that the ants begin to construct novel tracks by combining fruitful components of previously traversed trips. As more ants explore the laid-out trails, the promising sub-paths accumulate fresh pheromone and that in turn facilitates further innovation. In this way the ants eventually converge to an optimised passage which is composed of fragments that have the highest levels of pheromone deposits.
In broad terms, there are three main pillars to how ants find optimum routing. Firstly, they are keen explorers that record as well as share their trails with others via the secretion of pheromones. Secondly, ants routinely follow earlier expeditions whilst also being opportunistic in resolving path conflicts by switching to promising directions. Finally, the emergent outcome is that ants innovate incrementally by continually discovering new trails by merging efficient subparts of previous trips.
Overall, the behaviour displayed by ants is classified as an example of swarming which is a known characteristic of many types of insects, birds, and fish. It signifies a process of collectively solving compound problems in which individuals are constrained to make localised decisions with limited operational means at their disposal. A few more known instances of swarming are aggregate activities such as bird flocking as well as fish schooling.
As part of swarm behaviour, it is not difficult to understand how large combinations of simple actions lead to the resolution of complex situations. For example, let us imagine a hypothetical specie that can only perform two types of distinct operations namely attack, or defend. If we were to now consider two such beings working together, then their total number of responses increases from two to four as follows. So, they could both either choose to display combative intent or stay on their guards. Alternatively, they could swap roles by one of them becoming hostile and the other could defend. In general, as the size of the group increases, its sheer capacity to respond increases exponentially. For instance, as the cluster increases to say about twenty species, its list of potential reactions rises to a staggering one million combinations.
Therefore, when a group of entities working together is faced with a challenging situation, it has a better chance of success by virtue of having many options at responding. For instance, let us imagine a hostile scenario that is repeatedly faced by our hypothetical cohort. They could counter it with one of the many possible formations, however, some combinations may achieve better results than others. Hence, through the process of many years of trial and error, the group will eventually settle at a reaction that best combats that recurring condition. Therefore, despite being limited to just two actions, our imaginary specie can achieve high survival rates if its works in tandem with others to thwart an impending challenge.
Since last many years, scientists in the field of AI have found a fresh inspiration in the form of swarm behaviour to support the development of intelligent programs. Interestingly, this somewhat marks a shift from past approaches which was geared towards mimicking the human mind and its various faculties to create smart systems. Hence, the enhanced focus on swarming has led to the development of novel approaches to solve tough business problems. For example, the Ant Colony Optimisation (ACO) refers to an algorithm that imitates the process of ants jointly developing efficient routes during their foraging excursions. ACO has been put into operation to address difficult commercial situations involving complex routing, network configuration, load balancing, distribution, supply chain and logistics. Overall, this field is still in its infancy, however, as naturalists unravel more survival strategies of the animal kingdom, there is plenty of scope to adopt the same within AI systems.
Published in The Express Tribune, April 21st, 2021.
Like Opinion & Editorial on Facebook, follow @ETOpEd on Twitter to receive all updates on all our daily pieces.
COMMENTS
Comments are moderated and generally will be posted if they are on-topic and not abusive.
For more information, please see our Comments FAQ