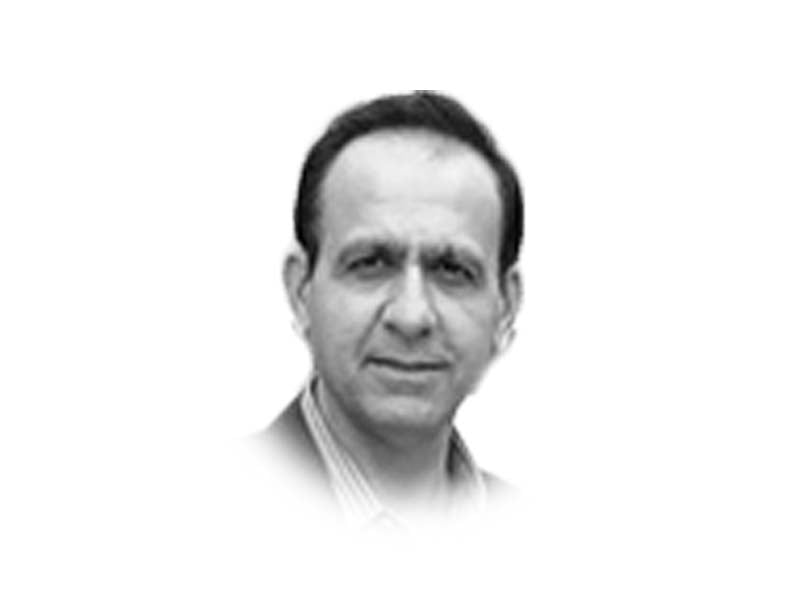
Optimal stopping approaches create a balance between what are called the exploration and the exploitation stages of matchmaking lifecycle. For example, in case of selling an item, the exploration phase implies receiving offers that help assess the market value without making a sale. This is followed by an exploitation phase in which the sale can be made based on offers that meet the expectations that were set during the exploration phase. If the exploration part is too short, we are likely to misunderstand the market and sell sub-optimally. On the other hand, if we unduly enhance the exploration phase, we risk missing out on current bids that might be the best that we could receive. Therefore, the main goal is to determine the most apt moment at which we should switch from an exploration to an exploitation stage. In simple terms, optimal stopping represents the required searching needed to gain a good measure of the market in order to subsequently make the best possible transaction.
To develop deeper understanding of how to compute optimal stopping point, mathematicians often refer to a puzzle called the game of googol which mimics real-life matchmaking scenarios. For readers who may not be familiar with the word googol, it is a very large number which is written as a 1 followed by 100 zeros. The game of googol starts with player one generating say 100 random numbers between 1 and googol. Those numbers are discretely written on a piece of paper and placed in front of us in the order in which they were generated. Our goal is to flip the slips one at a time and stop at the number that we believe is the highest amongst the set of 100 numbers. At any given time, the last number flipped is considered our pick as there is no reverting back to select previously revealed number. The catch of course is that if we view a number and decide not to stop and proceed with unveiling next numbers, there is a risk that the new numbers might be lower than the previous numbers.
We can easily map the above puzzle to the process of selling in which every flipped number represents an offer that is made to us for a hypothetical product. We must decide if we want to take up this offer or go to the next bid which is simulated by turning another slip of paper. Once an offer is rejected, it is classified as a missed opportunity and therefore we cannot go back to it. Hence, given any arbitrary state of the game with some known as well as hidden numbers, the goal is to maximise our chances to pick the highest in the list.
It turns out that mathematicians have analysed the game of google to come up with an optimal solution that is referred as the 37 percent rule. This means that the probability of making the best pick is highest if we would explore without stopping during the initial 37 numbers. After this stage, we would switch to exploitation phase by stopping at the very first number that is higher than the initial 37 numbers. Although this approach cannot guarantee the highest solution all the time, nevertheless, this strategy is scientifically proven to maximise our chances of achieving the best result.
To see how the 37 percent rule can be applied in practise, let us assume that you are a hiring manager who is evaluating prospects for a position in your team. If you have received 20 resumes of interested candidates, your chances of converging to the optimum will be highest if you were to interview the first 7 (37% of 20) applicants without making any decision. Next, you will need to transition to the exploitation phase and pick the very first interviewee that is better than the initial 7 contenders. If none of the remaining 13 prospects is better than the best from initial 7 applicants, then you would have to settle at the very last one in the list.
Although the 37 percent rule provides us with the optimum probability of choosing the highest number, it would still be classified as grossly optimistic approach since its performance on average or worst case could be poor. This is because with this strategy we are singularly aiming to achieve the ideal outcome and that is risky since we are ignoring situations when the probability does not work in our favour. In those conditions, we could really fall flat and converge to an extremely poor result. Hence, a more pragmatic approach would be to ensure that in case if we don’t achieve the optimum, we are at least settling on one of the better results in the list and thereby minimise the chances of catastrophic errors.
Fortunately, mathematicians have also worked out an effective stopping strategy for the pragmatic approach. In this case we would simply keep a count of turned numbers and the optimum transition from exploration to exploitation stage should happen when this count equals the square root of total size of the list. In summary, using our game of google example of 100 numbers, instead of switching after flipping 37 numbers, we would make the transition much earlier, that is after evaluating 10 numbers as that is the square root of 100.
Irrespective of whether you are seeking a pricey offer for your recently advertised car, or perhaps you are an eligible single looking for an ideal partner, the optimal stopping strategy has relevance for your quest to find a great match. Although it is a fact that finding the best match depends upon many factors beyond raw probabilities, however, by using scientific analysis in our assessment we can make a better selection resulting in minimizing later regret following such decisions.
COMMENTS
Comments are moderated and generally will be posted if they are on-topic and not abusive.
For more information, please see our Comments FAQ